
This means that to calculate the upper and lower bounds of the confidence interval, we can take the mean ☑.96 standard deviations from the mean. Example: Critical valueIn the TV-watching survey, there are more than 30 observations and the data follow an approximately normal distribution (bell curve), so we can use the z-distribution for our test statistics.įor a two-tailed 95% confidence interval, the alpha value is 0.025, and the corresponding critical value is 1.96. The author has included the confidence level and p-values for both one-tailed and two-tailed tests to help you find the t-value you need.įor normal distributions, like the t-distribution and z-distribution, the critical value is the same on either side of the mean. For the t-distribution, you need to know your degrees of freedom (sample size minus 1).Ĭheck out this set of t tables to find your t-statistic. The t-distribution follows the same shape as the z-distribution, but corrects for small sample sizes. If you are using a small dataset (n ≤ 30) that is approximately normally distributed, use the t-distribution instead. So if you use an alpha value of p 30) that is approximately normally distributed, you can use the z-distribution to find your critical values.įor a z-statistic, some of the most common values are shown in this table: Confidence level Your desired confidence level is usually one minus the alpha ( a ) value you used in your statistical test: For example, if you construct a confidence interval with a 95% confidence level, you are confident that 95 out of 100 times the estimate will fall between the upper and lower values specified by the confidence interval.

This is the range of values you expect your estimate to fall between if you redo your test, within a certain level of confidence.Ĭonfidence, in statistics, is another way to describe probability.
#STATA CONFIDENCE INTERVAL PLUS#
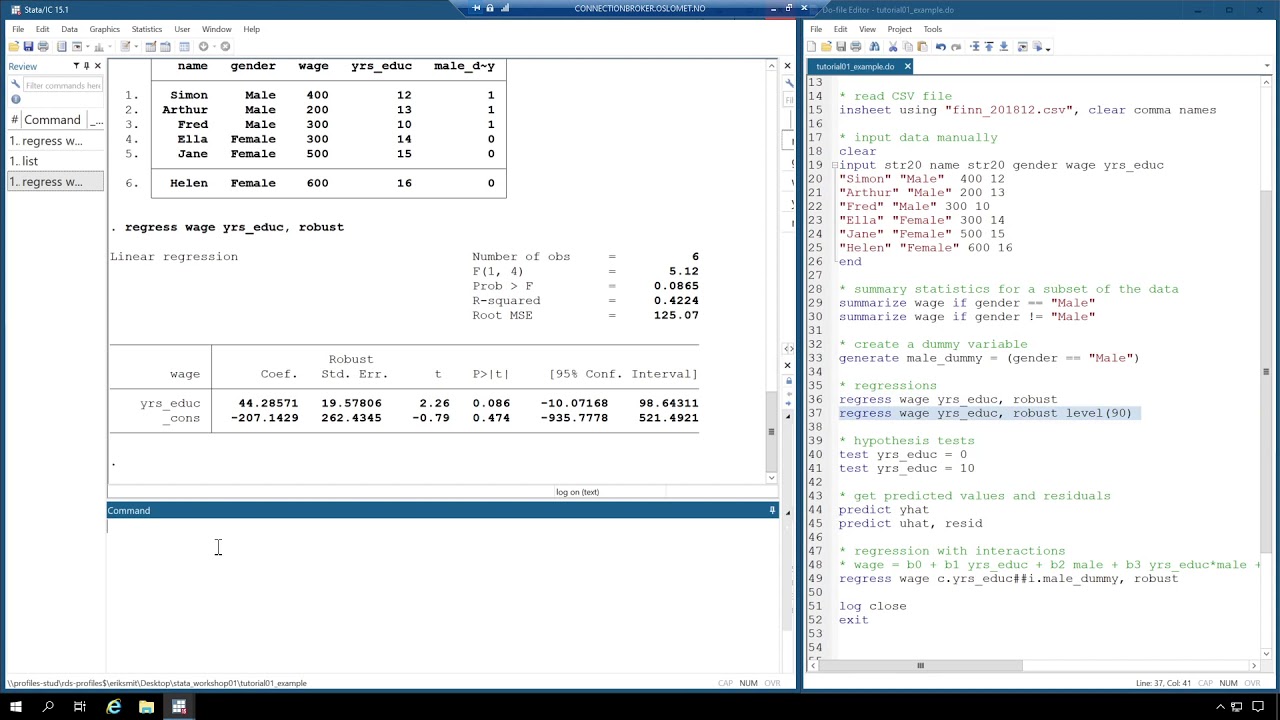
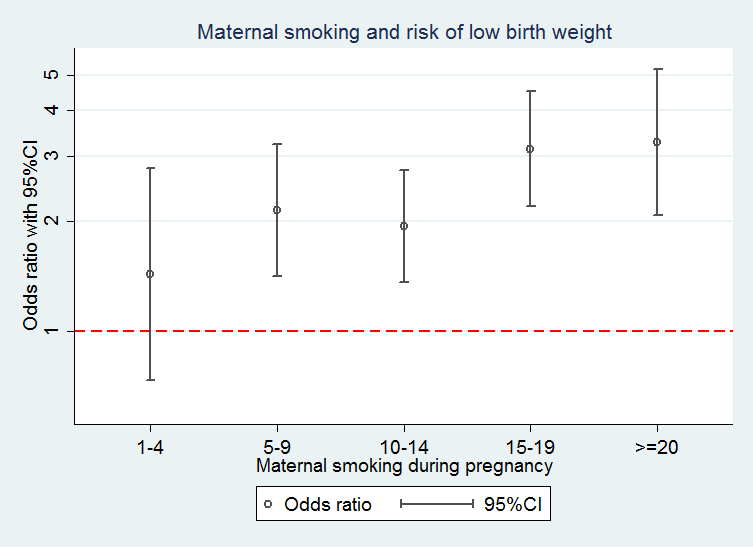
The three lines of the program do the following: We need the program to calculate the correlation, extract the value from r(rho), calculate the Fisher- \(z\) transformation, and return that value to r(). This makes the usual formula for a confidence interval, \(r \pm t_ \right) Without a transformation, the correlation coefficient is bounded in the interval \(\). We would like to also calculate the confidence interval for the correlation. The sample correlation coefficient between LSAT and GPA is 0.776. We want to estimate the correlation between LSAT and GPA scores. We have 15 paired observations of student LSAT scores and GPAs. The data used in this tutorial are from Efron and Tibshirani’s (1993) text on bootstrapping (page 19). See our blog post on bootstrapping for more specifics on the formulas used for the different types of bootstrap confidence intervals.
#STATA CONFIDENCE INTERVAL HOW TO#
This tutorial demonstrates how to use bootstrapping to calculate confidence intervals in Stata. The bootstrap is most commonly used to estimate confidence intervals. You can calculate a statistic of interest on each of the bootstrap samples and use these estimates to approximate the distribution of the statistic. The bootstrap is a statistical procedure that resamples a dataset (with replacement) to create many simulated samples.
